
DIY Tulle Tutu Table Skirt multicolor Tulle Tableware for Wedding Favors Birthday Party Decoration Home Textile|tulle tutu table skirt|table skirttutu table - AliExpress
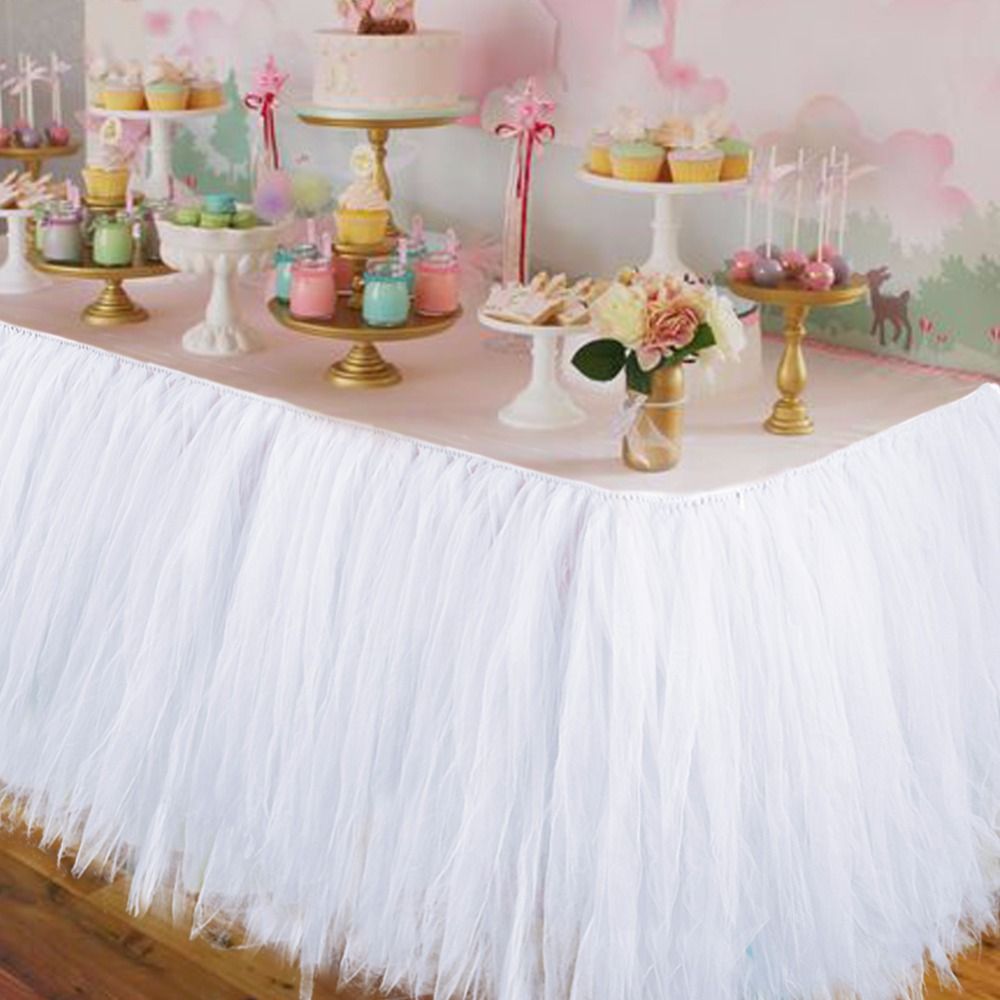
Wedding Party Tulle Tutu Gonna Tavolo Compleanno Baby Shower Decorazioni La Tavola Di Nozze Fai Da Te Articoli Artigianali Vendita Calda Da 29,15 € | DHgate Italy

100 centimetri x 80 centimetri Arcobaleno di Stile di Tulle Tutu Gonne Per Tavoli Da Tavola Per La cerimonia nuziale Del Partito Baby Shower Compleanno di Natale Decorazioni Da Tavola|tableware wedding|tableware partytableware
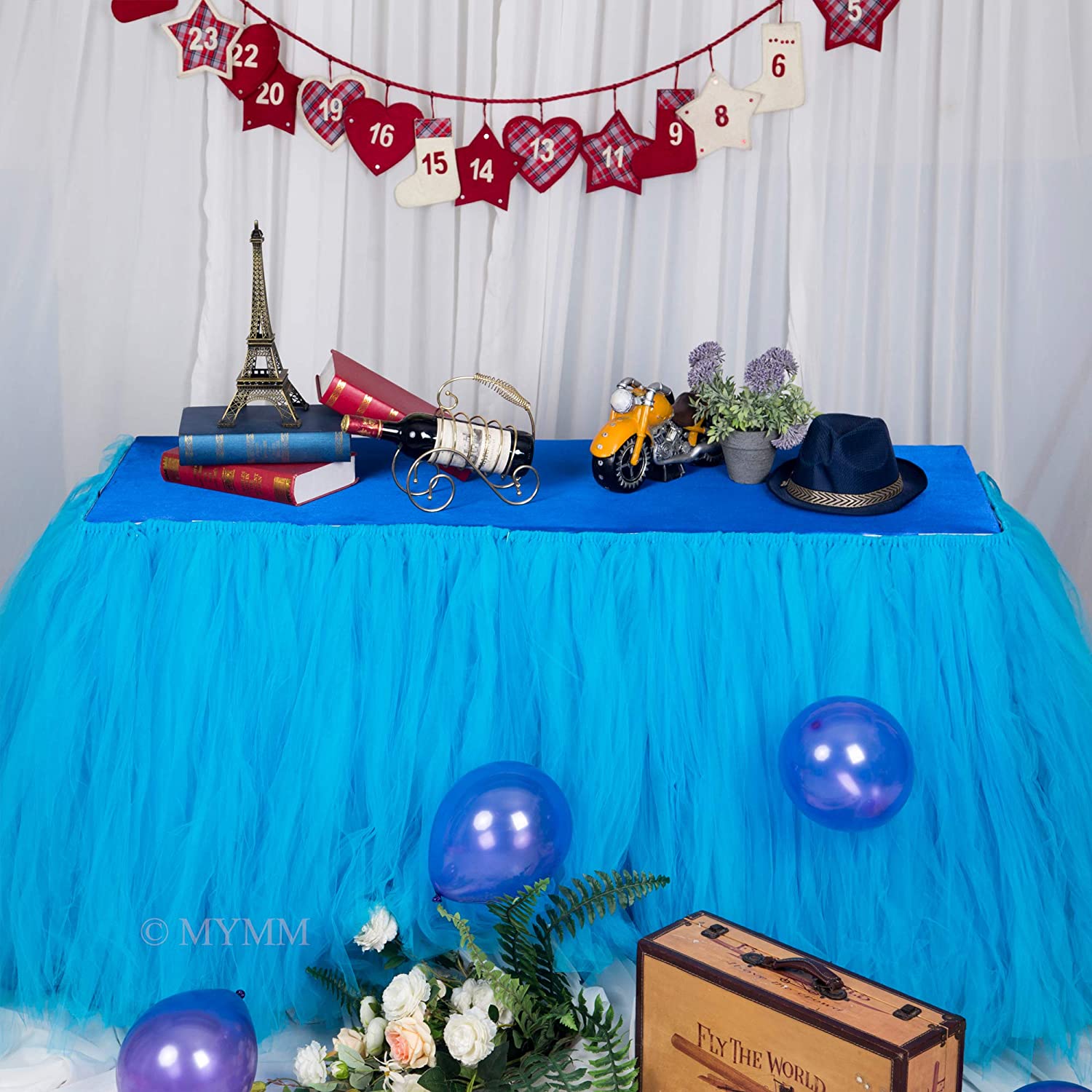
MYMM Gonna da Tavolo, Garza da scrivania in Tulle Romantico, Decorazione da tavola, tovaglia da Tavolo per Tutu con Fiocchi di Neve, per Baby Shower, Matrimonio, Compleanno,Natale - MYMM® Store IT

HBBMAGIC Bianco Blu Gonna da Tavolo in Tulle Bianco tutù Gonne da Tavolo per Festa di Nozze Festa di Compleanno Decorazione Baby Shower, L183 × H76cm Gonne da Tavolo Fai da Te :

Gonna da tavolo in tulle per banchetto nuziale, decorazione casalinga, feste di Natale Pink: Amazon.it: Casa e cucina | Gonne, Tavolo tulle, Tulle
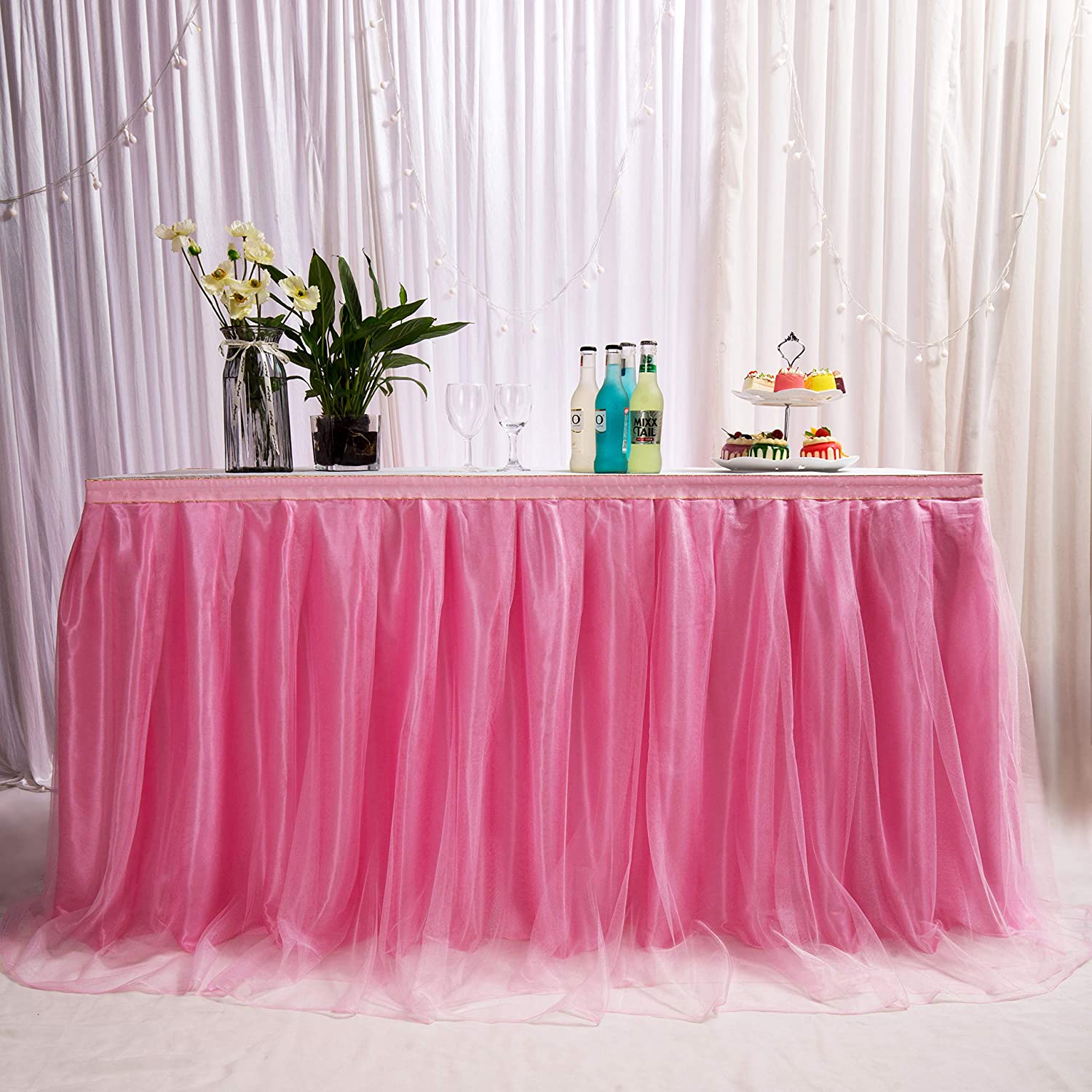
MYMM Gonna da Tavolo, Garza da scrivania in Tulle Romantico, Decorazione da tavola, tovaglia da Tavolo per Tutu con Fiocchi di Neve, per Baby Shower, Matrimonio, Compleanno,Natale - MYMM® Store IT

DishyKooker Gonna da tavolo in morbido tulle tutù, 91,4 x 78,7 cm, con adesivo magico, tovaglia in tulle per feste, matrimoni, baby shower e decorazioni per la casa rosa : Amazon.it: Casa
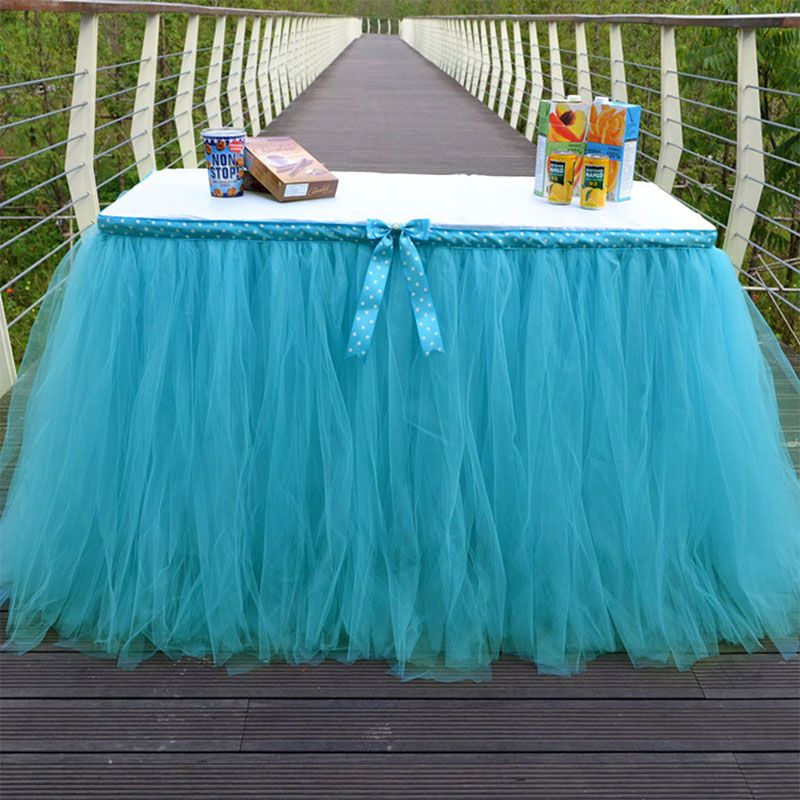
1 PZ Dot Bow Bow Tulle Gonna Tavolo Fai Da Te Tutu Stoviglie Gonne La Cerimonia Nuziale Festa Di Compleanno Decorazione Baby Shower Favori 91.5 * 80 Cm Da 30,41 € | DHgate Italy
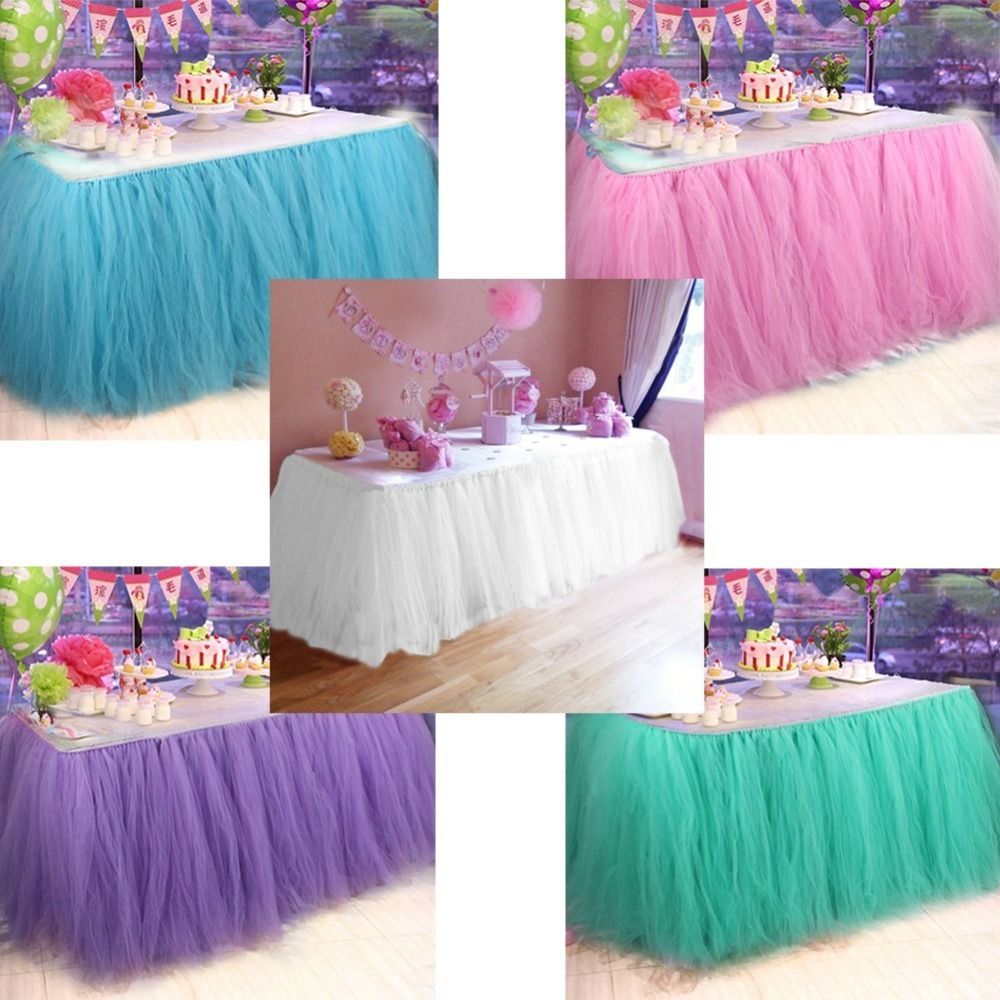
Ourwarm Fai Da Te Tavolo Battiscopa Personalizza Handmade Tulle Tutu Gonna Tavolo Festa Di Compleanno Decorazione Di Cerimonia Nuziale Tessili La Casa Q190430 Da 13,18 € | DHgate Italy

AMOYER Tulle Tutu Tabella Gonna Stoviglie Tovaglia per Halloween Theme Partito Decorazioni di Favore Hobby creativi Articoli per feste e compleanni berjaya.edu.my

HBBMAGIC Bianco LED Gonna da Tavolo in Tulle tutù Gonne da Tavolo per Festa di Nozze Festa di Compleanno Decorazione Baby Shower, L183 × H76cm Gonne da Tavolo Fai da Te :

NSSONBEN Azzurro Tulle Gonna da Tavolo Tutu Gonne da Tavolo per Feste Matrimoni Festa di Compleanno Bambino Doccia Decorazione 3Yard/9ft/L275cm*H77cm : Amazon.it: Casa e cucina
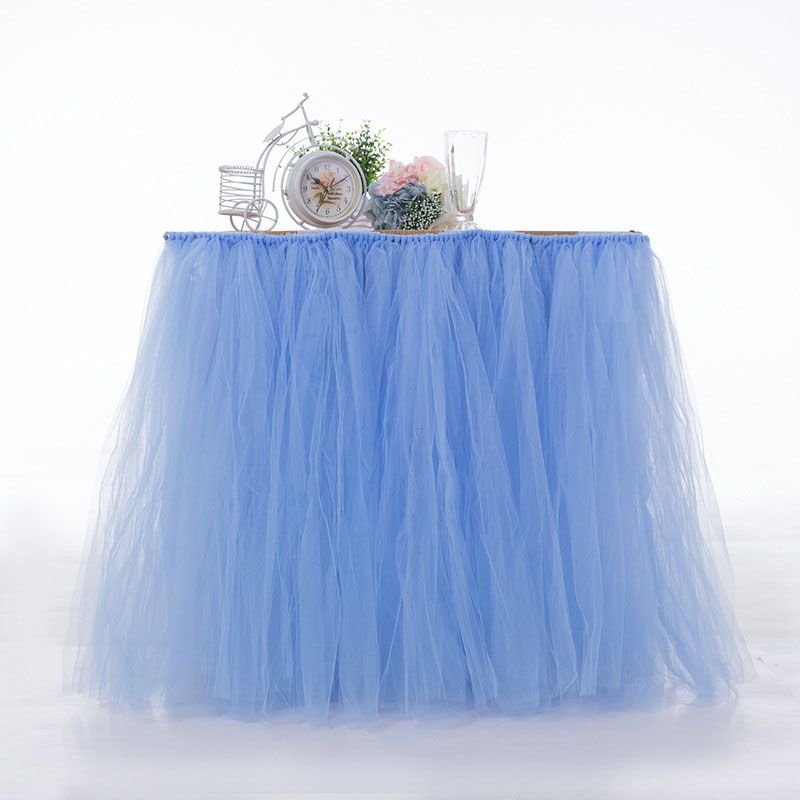
Wedding Party Tulle Tutu Gonna Tavolo Compleanno Baby Shower Decorazioni La Tavola Di Nozze Fai Da Te Articoli Artigianali Vendita Calda Da 21,98 € | DHgate Italy

Gonna da tavolo tutu gonna da tavolo in Tulle - Etsy Italia | Wedding table, Wedding party decorations diy, Wedding ceiling decorations
